How do we predict where wheat blast disease might cause infections?
Model background
Wheat blast (Magnaporthe oryzae Pathotype Triticum (MoT) can be a particularly devastating disease of wheat (Figure 1). A simulation model that accounts for meteorological conditions and their effect on inoculum build-up and infection was developed in Brazil, where wheat blast has been a problem for over 30 years (Fernandes et al., 2017). This predictive model was developed and evaluated based on the analysis of historical epidemics and weather series data in the northern Paraná state in Brazil and then applied to Bangladesh. Expert national epidemiological knowledge of the meteorological conditions during which infections occurred in both countries were also employed in model parameterization.
Importantly, this model also assumes the geographically uniform presence of MoT inoculum in the environment for which simulations are run. In other words, the model assumes that inoculum is uniformly present throughout locations that it predicts for, and does not yet account for source-sink and spore dispersal mechanisms. The disease incidence and hourly-scale weather datasets examined by Fernandes et al., (2017) for Brazil encompassed the 2001–2012 period.
We utilized a specific database management application (Farman et al., 2017) developed using R Shiny. This application was programmed to visualize and identify patterns in weather variables during two major MoT outbreaks (2004 and 2009) in Brazil. Uncommonly humid and warm weather was observed for most locations during a 60-day period preceding wheat heading during both major outbreak years. These conditions were therefore assumed to be key drivers of inoculum build-up and airborne spores from inoculum sources in surrounding regions. Using this as the basis for prediction, we transferred this wheat blast ‘early warning’ system to Bangladesh, where the disease was found for the first time in 2016 (Malaker et al. 2016; Figure 2).
Model inputs
The wheat blast predictive model is driven by weather forecast information and can be validated with historical (observed) weather data. In the case of Brazil, hourly weather data for the state of Paraná, Brazil is collected from the SIMEPAR (Technological Institute Simepar) automated weather station network. The automated weather station network from INMET (National Institute of Meteorology) also provide hourly weather data for the whole country. The short-term numerical weather forecast used for predictions of blast infection for Brazil is provided by INPE (National Institute of Space Research) in a grid of 15 x 15 km. These are used for driving five-day forecasts of wheat blast disease risks.
In Bangladesh, observed three-hourly historical weather data has been kindly supplied from the BMD (Bangladesh Meteorological Department) automated weather station network and a small network of automatic hourly reporting weather stations maintained by the International Maize and Wheat Improvement Center (CIMMYT). Where observations are not hourly, as in the case of the BMD stations, data were interpolated to provide hourly information. For reasons of simplification, hourly values were linearly interpolated. In addition, a five-day numerical weather forecast for Bangladesh is an output of the Weather Research and Forecasting (WRF) Model provided by BMD in a grid of 17 x 17 km to drive early warning forecasts. In addition users can also explore the implications of weather forecasts provided by the Dark Sky Company, LLC to drive the wheat blast model in Bangladesh.
Model configuration
The wheat blast prediction model which forms the basis of our wheat blast disease early warning system has four components. The first component assumes sporulative wheat blast lesions are present in the environment in which prediction is made and estimates the rate of conidiophore development as a function of temperature and relative humidity. These model conditions are based on prior work conducted by Bregaglio and Donatelli (2015) in their work on rice blast disease. Both temperature and relative humidity are integrated to estimate blast inoculum potential by solving equation 1 for the hourly sum of inoculum potential (IP) over the cropping season.
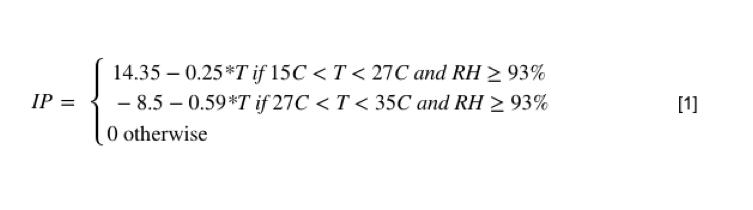
In Equation 1, T and RH refer to air temperature and relative humidity, respectively. Where RH is below the threshold in Equation 1, the model does not accumulate thermal time, and as such it does not simulate individual MoT spore development. Conversely, the model does calculate the development of a spore cloud and the density of spores in a 1 m3 area over the crop canopy, subject to assumptions of air current uptake, atmospheric diffusion and wind shear, all of which can affect and reduce spore longevity (Mousanejad et al. 2009). Survival of spores while airborne may also be affected by temperature, solar and ultraviolet radiation, in addition to relative humidity (Deacon, 2005). Based on this literature, we applied a simple metric and assumed that spore cohorts have a half-life of three days within any seven-day window. For each 24 period during which these conditions were met, the model accumulates values that result in a blast spore ‘index’ that is used to estimate the risk of outbreak. The model also determines the number and timing of days with climatic conditions favoring blast infection using a conditional ruleset. Days favoring infection (DFI) are consequently declared following spore cloud development when the daily maximum temperature exceeded 23°C, and when temperature amplitude (calculated as the daily minimum temperature subtracted from daily maximum temperature) was > 15°C, with mean daily RH above 93%, as in Equation 1. Rain can also damage conidiophore and/or was freshly deposited spores off of leaves and wheat spikes (Mousanejad et al. 2009; Suzuki (1975). If > 5mm of rain is accumulated within a 24-hour period, DFI is set to non-favorable.
Early warning generation
The model simulation that drives the early warning system and disease alerts start at the beginning of the wheat season. The model assumes that inoculum is present in the environment and that it originates from host plant tissues. These include wheat, but could also include secondary hosts such as weeds. For each grid call found the maps of Bangladesh or Brazil, the model simulates the number of spores that are present in the air. Spore development in turn is expressed in density the amount of inoculum that builds above the crop canopy during the wheat vegetative growth period. In the spore cloud, the predicted density of spores per cubic meter was conservatively classified as high (> 8000 m -3), moderate (> 3000 m-3 and < 8000 m-3), and low ( < 3000 m-3). Based on these densities, it is important to note here that the forecast model does not provide early warnings for infection alone. Rather, it provides early warnings when there is a risk of disease outbreak based on the density of spores simulated and suitable environmental conditions for infection to result in outbreak.
The grids in green on the maps represent no risk while yellow and red squares indicate, respectively, moderate and high risk. A new risk map is available for each of the five days forecasted, with early warnings delivered by email or other mechanisms to subscribed users for each day in which risk is observed for selected specific locations, as based on model grid run outputs.
The outputs from the model is a map of specific states within Brazil, or for the whole of Bangladesh, for the wheat growing areas in Bangladesh that presents the risk of wheat crop infection for each grid cell for each day of ingested weather forecast data. Following infection, it generally takes around 10 days before disease lesions become available to the naked eye (Bregaglio et al. 2016). Note that in the case of Bangladesh, users have the choice of switching a toggle button to examine disease risks for the whole country, or for areas deemed by the Bangladesh Agricultural Research Council as suitable for wheat production (BARC 2020). The resulting model adequately described DFIs during observed epidemic and non-epidemics years during and beyond the study period in Brazil, and appears to adequately simulate the 2016 outbreak in Bangladesh.
Early warning advisory delivery and wheat phenology in Bangladesh
In Bangladesh, advisories are pushed to extension agents and farmers via email and other mechanisms when a day favoring infection risk is identified for specific locations selected by users as of interest. Early warnings are constrained to the period from January through 4 April, corresponding to the potential times when farmers’ wheat crops in Bangladesh could be between heading and maturity, as the key phenological stages during which the crop is at risk of yield loss due to infection. A matrix of potential advisory message structures for the winter ‘rabi’ season wheat crop was developed to facilitate this process (Table 1).
Table 1. Wheat blast disease risks and advisory message structure for Bangladesh
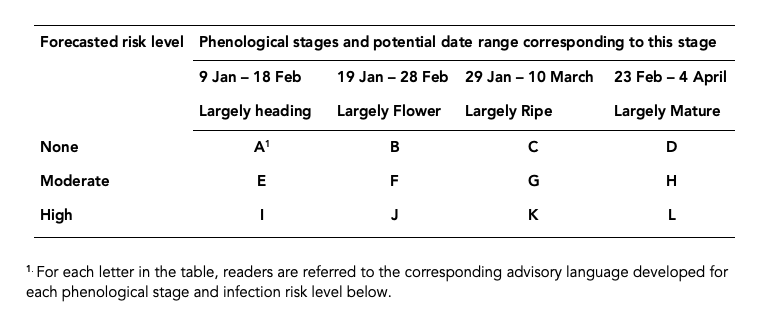
- The crop is largely at heading and the date is between 9 January to 18 February. No risk of disease outbreak. No advisory is emailed or provided.
- The crop is largely at flowering and the date is between 19 January to 28 February. No risk of disease outbreak. No advisory is emailed or provided.
- The crop is largely ripe, and the date is between 29 January to 10 March. No risk of disease outbreak. No advisory is emailed or provided.
- The crop is largely ripe, and the date is between 23 February to 4 April. No risk of disease outbreak. No advisory is emailed or provided.
-
The crop is largely at heading and the date is between 9 January to 18 February. Advisory language structure:
You are receiving this advisory because nighttime temperatures have recently been above 15°C and it has been relatively humid. These conditions favor wheat blast disease infection. If wheat is close to or at heading, and farmers have not yet applied fungicides, then you may wish to advise farmers to act in the next few days to prevent infection.
Farmers should use officially recommend registered fungicide practices given in the link here. Remember to advise farmers not to use the same fungicide more than one time during a crop. Fungicides are poison and they should be only applied by experienced applicators trained in use of fungicides while wearing gloves, a mask, gum boots, rubber pants and coveralls. Advice on how to prepare and apply fungicides can be found by clicking here.
Farmers are also advised to grow wheat blast resistant varieties such as BARI Gom 33 and to plant their fields as early as possible. This will reduce risk of disease and improve yield.
-
The crop is largely at flowering and the date is between 19 January to 28 February. Advisory language structure:
You are receiving this advisory because nighttime temperatures have recently been above 15°C and it has been relatively humid. These conditions favor wheat blast disease infection. If wheat is at heading or flowering, and farmers have not yet applied fungicides, then you may wish to advise farmers to act in the next few days to prevent infection.
Farmers should use officially recommend registered fungicide practices given in the link here. Remember to advise farmers not to use the same fungicide more than one time during a crop. Fungicides are poison and they should be only applied by experienced applicators trained in use of fungicides while wearing gloves, a mask, gum boots, rubber pants and coveralls. Advice on how to prepare and apply fungicides can be found by clicking here.
Farmers are also advised to grow wheat blast resistant varieties such as BARI Gom 33 and to plant their fields as early as possible. This will reduce risk of disease and improve yield.
-
The crop is largely ripe, and the date is between 29 January to 10 March. Advisory language structure:
You are receiving this advisory because nighttime temperatures have recently been above 15°C and it has been relatively humid. These conditions favor wheat blast disease infection. If wheat is at flowering and farmers have not yet applied fungicides, then you may wish to advise farmers to act in the next few days to prevent infection.
Farmers should use officially recommend registered fungicide practices given in the link here. Remember to advise farmers not to use the same fungicide more than one time during a crop. Fungicides are poison and they should be only applied by experienced applicators trained in use of fungicides while wearing gloves, a mask, gum boots, rubber pants and coveralls. Advice on how to prepare and apply fungicides can be found by clicking here. If wheat is at ripening, then fungicides are not. advised.
Farmers are also advised to grow wheat blast resistant varieties such as BARI Gom 33 and to plant their fields as early as possible. This will reduce risk of disease and improve yield.
- The crop is largely ripe, and the date is between 23 February to 4 April. No risk of disease outbreak. No advisory is emailed or provided.
-
The crop is largely at heading and the date is between 9 January to 18 February. Advisory language structure:
You are receiving this advisory because nighttime temperatures have recently been above 15°C and it has been relatively humid. These conditions favor wheat blast disease infection. If wheat is close to or at heading, and farmers have not yet applied fungicides, then you may wish to advise farmers to act immediately to avoid risk of disease outbreak.
Farmers should use officially recommend registered fungicide practices given in the link here. Remember to advise farmers not to use the same fungicide more than one time during a crop. Fungicides are poison and they should be only applied by experienced applicators trained in use of fungicides while wearing gloves, a mask, gum boots, rubber pants and coveralls. Advice on how to prepare and apply fungicides can be found by clicking here.
Farmers are also advised to grow wheat blast resistant varieties such as BARI Gom 33 and to plant their fields as early as possible. This will reduce risk of disease and improve yield.
-
The crop is largely at flowering and the date is between 19 January to 28 February. Advisory language structure:
You are receiving this advisory because nighttime temperatures have recently been above 15°C and it has been relatively humid. These conditions favor wheat blast disease infection. If wheat is at heading or flowering, and farmers have not yet applied fungicides, then you may wish to advise farmers to act immediately to avoid risk of disease outbreak.
Farmers should use officially recommend registered fungicide practices given in the link here.Remember to advise farmers not to use the same fungicide more than one time during a crop. Fungicides are poison and they should be only applied by experienced applicators trained in use of fungicides while wearing gloves, a mask, gum boots, rubber pants and coveralls. Advice on how to prepare and apply fungicides can be found by clicking here.
Farmers are also advised to grow wheat blast resistant varieties such as BARI Gom 33 and to plant their fields as early as possible. This will reduce risk of disease and improve yield.
-
The crop is largely ripe, and the date is between 29 January to 10 March. Advisory language structure:
You are receiving this advisory because nighttime temperatures have recently been above 15°C and it has been relatively humid. These conditions favor wheat blast disease infection. If wheat is at flowering or ripening and farmers have not yet applied fungicides, then you may wish to advise farmers to act immediately to avoid risk of disease outbreak.
Farmers should use officially recommend registered fungicide practices given in the link here. Remember to advise farmers not to use the same fungicide more than one time during a crop. Fungicides are poison and they should be only applied by experienced applicators trained in use of fungicides while wearing gloves, a mask, gum boots, rubber pants and coveralls. Advice on how to prepare and apply fungicides can be found by clicking here. If wheat is at ripening, then fungicides are not advised.
Farmers are also advised to grow wheat blast resistant varieties such as BARI Gom 33 and to plant their fields as early as possible. This will reduce risk of disease and improve yield.
- The crop is largely ripe, and the date is between 23 February to 4 April.As the wheat crop is likely to be largely mature, the risk of disease outbreak. No advisory is emailed or provided.
References
BARC. (2020) Bangladesh Agricultural Research Council (BARC) Crop Zoning Maps. Available online: http://cropzoning.barcapps.gov.bd/homes/downloads/2. Verified February 2, 2020.
Bregaglio S, Donatelli M (2015) A set of software components for the simulation of plant airborne diseases. Environ Model Softw 72:426–444.
Bregaglio, S., Titone, P., Cappelli, G., Tamborini, L., Mongiano, G., & Confalonieri, R. (2016). Coupling a generic disease model to the WARM rice simulator to assess leaf and panicle blast impacts in a temperate climate. European Journal of Agronomy, 76, 107–117. https://doi.org/10.1016/j.eja.2016.02.009.
Deacon, J. (2005) Fungal spores, spore dormancy, and spore dispersal, in fungal biology, 4th edition, Blackwell doi:10.1002/9781118685068.Chapter 10.
Farman M., Peterson G.L., Chen L., Starnes J.H., Valent B., Bachi M.P., Murdock L., Hershman D.E., Pedley K.F., Fernandes J.M.C., Bavaresco J. (2017). The Lolium pathotype of Magnaporthe oryzae recovered from a single blasted wheat plant in the United States. Plant Dis. 101:684–692.
Fernandes, J. M. C., Nicolau, M., Pavan, W., Hölbig, C. A., Karrei, M., de Vargas, F., Tsukahara, R. Y. (2017). A weather-based model for predicting early season inoculum build-up and spike infection by the wheat blast pathogen. Trop. Plant Pathology. https://doi.org/10.1007/s40858-017-0164-2.
Malaker, P. K., Barma, N. C. D., Tewari, T. P., Collis, W. J., Duveiller, E., Singh, P. K., Joshi, A. K., Singh, R. P., Braun, H.-J., Peterson, G. L., Pedley, K. F., Farman, M. and Valent, B. (2016). First Report of Wheat Blast Caused by Magnaporthe oryzae Pathotype Triticum in Bangladesh. Plant Dis. 100:2330.
Mousanejad, S., Alizadeh, A., and Safaie, N. (2009). Effect of weather factors on spore population dynamics of rice blast fungus in Guilan province. J. of Plant Prot. Res. 49:319-329.
Suzuki, H. (1975). Meteorological Factors in the Epidemiology of Rice Blast. Ann. Rev. Phytopathology 13:239-256.